Machine Learning and Tactical Asset Allocation – Part II: Decomposing the Machine Learning Signals
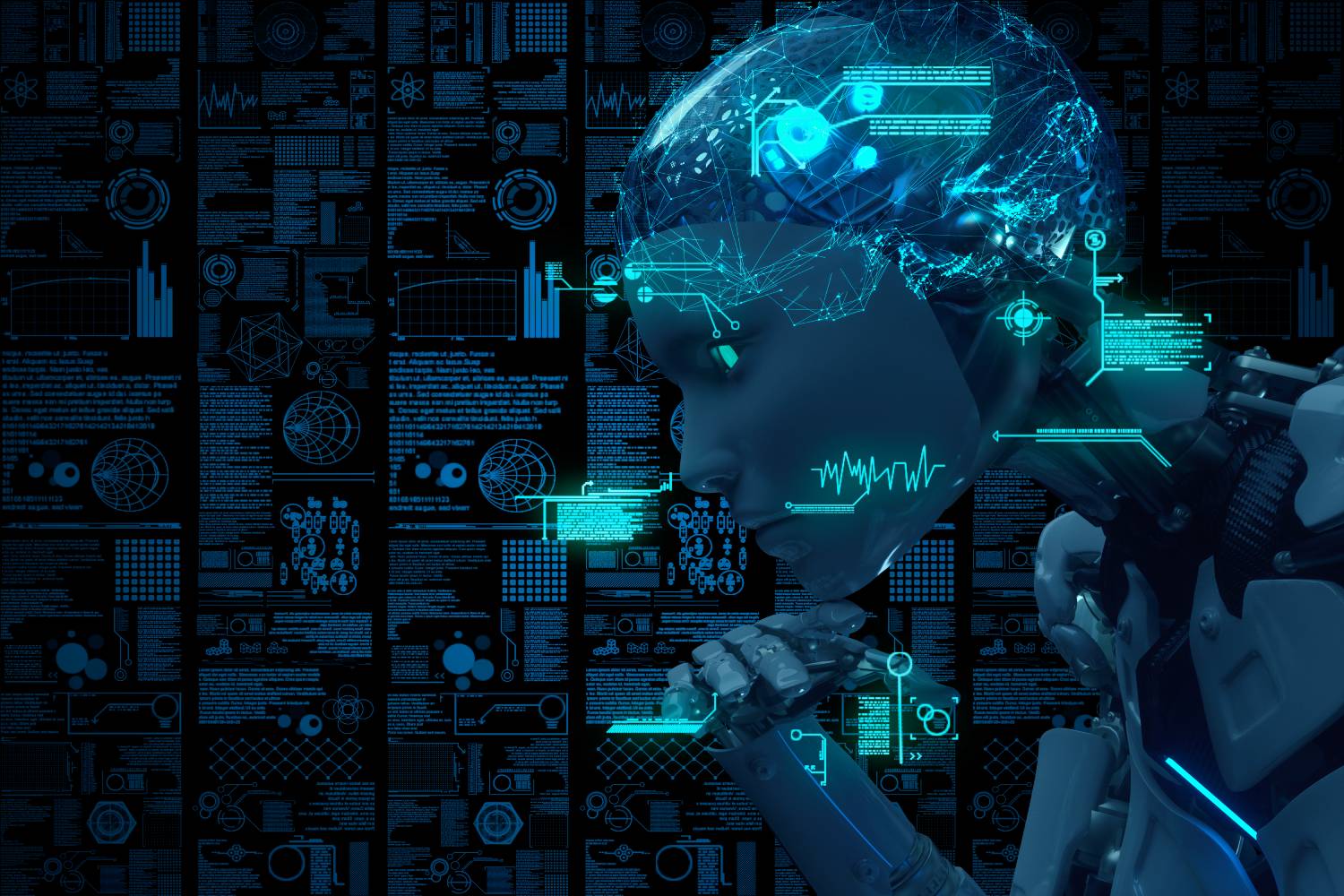
The latest views, research and investment insights from the experts of our ecosystem
© Generali Investments, all rights reserved. This website is provided by Generali Investments and is considered as a marketing communication and financial promotion related to the products and services of the following companies belonging to the Generali group: Generali Investments Partners S.p.A. Società di gestione del risparmio, Generali Insurance Asset Management S.p.A. Società di gestione del risparmio, Generali Investments Luxembourg S.A. and Generali Investments Holding S.p.A. (hereinafter jointly, Generali Investments). Moreover, the website may contain marketing communication and financial promotion of products and services of companies part of the multi-boutique platform coordinated by Generali Investments Partners S.p.A. Società di gestione del risparmio, and in particular of Infranity, Sycomore Asset Management, Aperture Investors LLC., Plenisfer Investments SGR, Lumyna Investments, Sosteneo Infrastructure Partners SGR, Axis Retail Partners S.p.A. and Generali Real Estate S.p.A. Società di Gestione del Risparmio